CCTV-Pipe Dataset
Abstract
We have carefully collected and annotated two new industrial video datasets, namely QV-Pipe and CCTV-Pipe, for video understanding in urban pipe inspection. Specifically, QV-Pipe is used for video defect classification (Task1) and CCTV-Pipe is used for temporal defect localization (Task 2).
Note that, all the participants are required to sign a copyright form for academic research, before getting our datasets. Besides, the datasets are based on the real-world pipe networks. Hence, we have deleted the information of street, city and any other about privacy in our datasets.
Data Collection & Annotation
Our CCTV-Pipe dataset consists of 16 defect categories including structural and functional defects in the pipe. It contains 575 videos with 87 hours, which are collected from real-world urban pipe systems. Different from traditional temporal action localization, our goal in this realistic scenario is to find preferable temporal locations of defects from a untrimmed CCTV video, instead of exact temporal boundaries. Hence, the professional engineers are asked to annotate a single frame for each defect. The annotation procedure has been checked multiple rounds with cross validation, to guarantee label quality.
Data Comparison
We show some examples of CCTV-Pipe in Figure 1. We can see that, several defects appear at the same temporal location. Additionally, as demonstrated in Figure 2, the number of defects in each category ranges from 8 to 2,770. Such long-tailed distribution also raises new challenges for temporal defect localization.
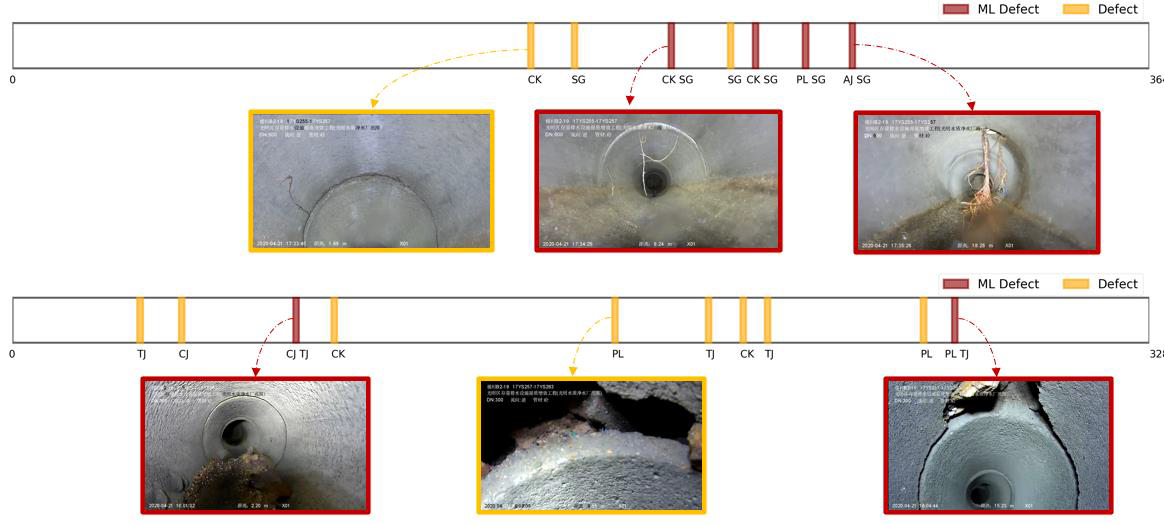
Figure 1. Examples of Our CCTV-Pipe Dataset. (ML: Multi-Labeled)
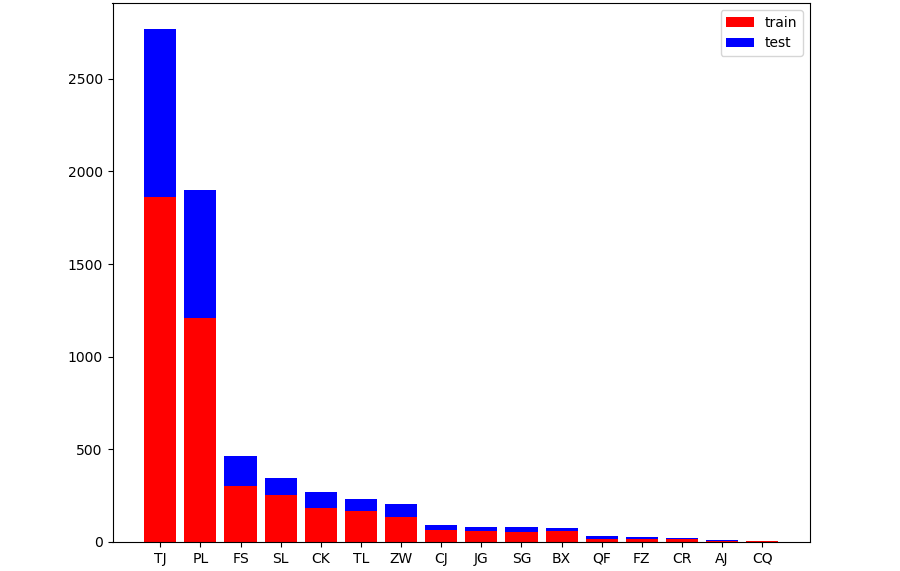
Figure 2. Data Distribution of CCTV-Pipe
Moreover, we compare it with the existing video benchmarks in temporal localization. As shown in Table 1, our CCTV-Pipe dataset shows the following distinct characteristics. First, compared to the existing benchmarks, videos in our CCTV-Pipe can be very long in practice, e.g., average video duration is 545 s. It is quite challenging to find temporal locations of pipe defect from such long untrimmed videos. Second, instead of traditional segment annotation, we adopt single-frame annotation for realistic demand in urban pipe inspection. Moreover, multiple defects can densely appear at the same temporal location. These facts make our CCTV-Pipe as a challenging dataset for temporal localization.
Table 1. Temporal Localization Benchmark Comparison
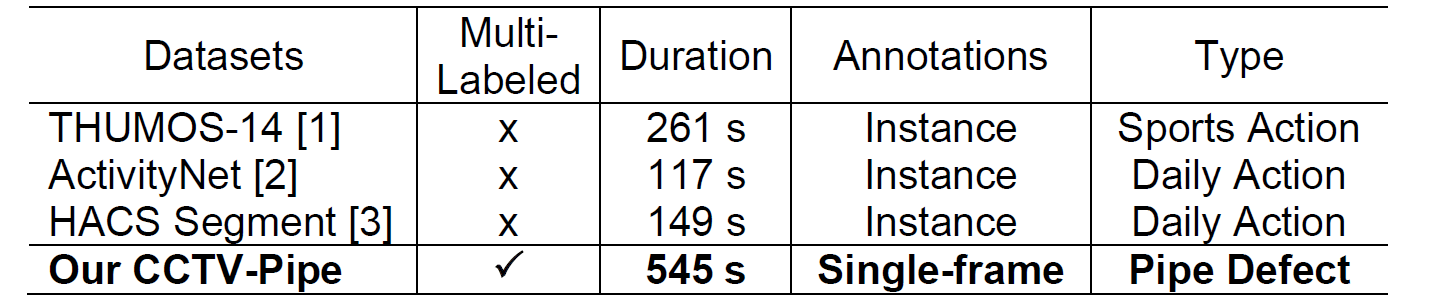
Finally, we compare it with the existing benchmarks in pipe defect inspection. As shown in Table 2, our dataset is based on videos, which is closer to urban pipe inspection in the real scenes. Moreover, our dataset is much larger than the existing ones, which opens new opportunities to develop powerful models for automatic defect inspection of urban pipe systems.
Table 2. Urban Pipe Inspection Dataset Comparison
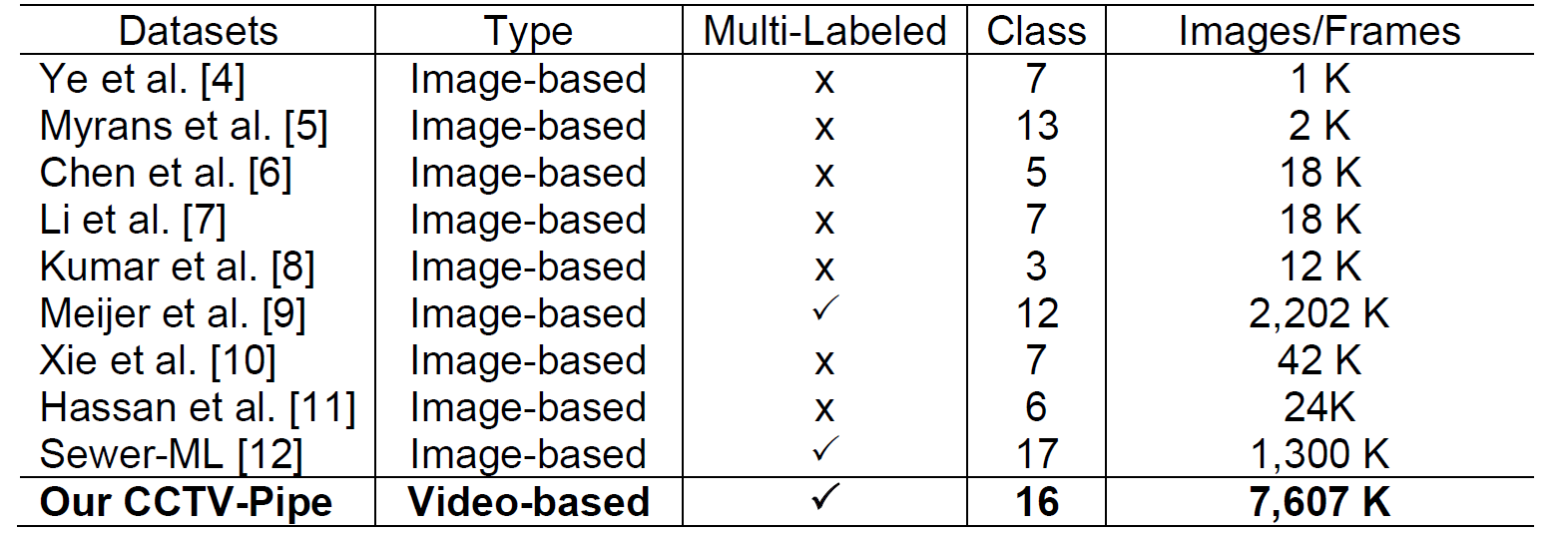
Download
Please refer to the competition page for more information.
Contact Us : Yi Liu ( yi.liu1@siat.ac.cn )
Reference
- [1] Idrees H, Zamir A R, Jiang Y G, et al. The THUMOS challenge on action recognition for videos “in the wild”. Computer Vision and Image Understanding, 155, 2017.
- [2] Caba Heilbron F, Escorcia V, Ghanem B, et al. Activitynet: A large-scale video benchmark for human activity understanding. IEEE conference on computer vision and pattern recognition. 2015.
- [3] Zhao H, Torralba A, Torresani L, et al. Hacs: Human action clips and segments dataset for recognition and temporal localization. IEEE/CVF International Conference on Computer Vision. 2019.
- [4] Xiangyang Ye, Jian’e Zuo, Ruohan Li, Yajiao Wang, Lili Gan, Zhonghan Yu, and Xiaoqing Hu. Diagnosis of sewer pipe defects on image recognition of multi-features and support vector machine in a southern chinese city. Frontiers of Environmental Science & Engineering, 13(2), 2019.
- [5] Joshua Myrans, Richard Everson, and Zoran Kapelan. Automated detection of fault types in cctv sewer surveys. Journal of Hydroinformatics, 21(1):153–163, 2018.
- [6] Kefan Chen, Hong Hu, Chaozhan Chen, Long Chen, and Caiying He. An intelligent sewer defect detection method based on convolutional neural network. IEEE International Conference on Information and Automation, 2018.
- [7] Duanshun Li, Anran Cong, and Shuai Guo. Sewer damage detection from imbalanced cctv inspection data using deep convolutional neural networks with hierarchical classification. Automation in Construction, 101, 2019.
- [8] Srinath S. Kumar, Dulcy M. Abraham, Mohammad R. Jahanshahi, Tom Iseley, and Justin Starr. Automated defect classification in sewer closed circuit television inspections using deep convolutional neural networks. Automation in Construction, 91, 2018.
- [9] Dirk Meijer, Lisa Scholten, Francois Clemens, and Arno Knobbe. A defect classification methodology for sewer image sets with convolutional neural networks. Automation in Construction, 104, 2019.
- [10] Qian Xie, Dawei Li, Jinxuan Xu, Zhenghao Yu, and Jun Wang. Automatic detection and classification of sewer defects via hierarchical deep learning. IEEE Transactions on Automation Science and Engineering, 2019.
- [11] Syed Ibrahim Hassan, L. Minh Dang, Irfan Mehmood, Suhyeon Im, Changho Choi, Jaemo Kang, Young-Soo Park, and Hyeonjoon Moon. Underground sewer pipe condition assessment based on convolutional neural networks. Automation in Construction, 106, 2019.
- [12] Haurum J B, Moeslund T B. Sewer-ML: A Multi-Label Sewer Defect Classification Dataset and Benchmark. IEEE/CVF Conference on Computer Vision and Pattern Recognition. 2021: 13456-13467.