QV-Pipe Dataset
Abstract
We have carefully collected and annotated two new industrial video datasets, namely QV-Pipe and CCTV-Pipe, for video understanding in urban pipe inspection. Specifically, QV-Pipe is used for video defect classification (Task1) and CCTV-Pipe is used for temporal defect localization (Task 2).
Note that, all the participants are required to sign a copyright form for academic research, before getting our datasets. Besides, the datasets are based on the real-world pipe networks. Hence, we have deleted the information of street, city and any other about privacy in our datasets.
Data Collection & Annotation
The QV-Pipe dataset consists of 1 normal class and 16 defect classes and 9.6k videos, which are collected from real-world urban pipes and annotated by professional engineers. The total duration of all videos exceeds 55 hours. Because the pipe situation is complex, multiple defects often appear at the same time, so each video is annotated by multiple labels. To obtain accurate annotations of defect instances, professional engineers are asked to check all the videos multiple rounds with cross validation. Given a QV video, our goal is to predict multiple labels of pipe defects in this video. Examples of QV-Pipe are shown in Figure 1.
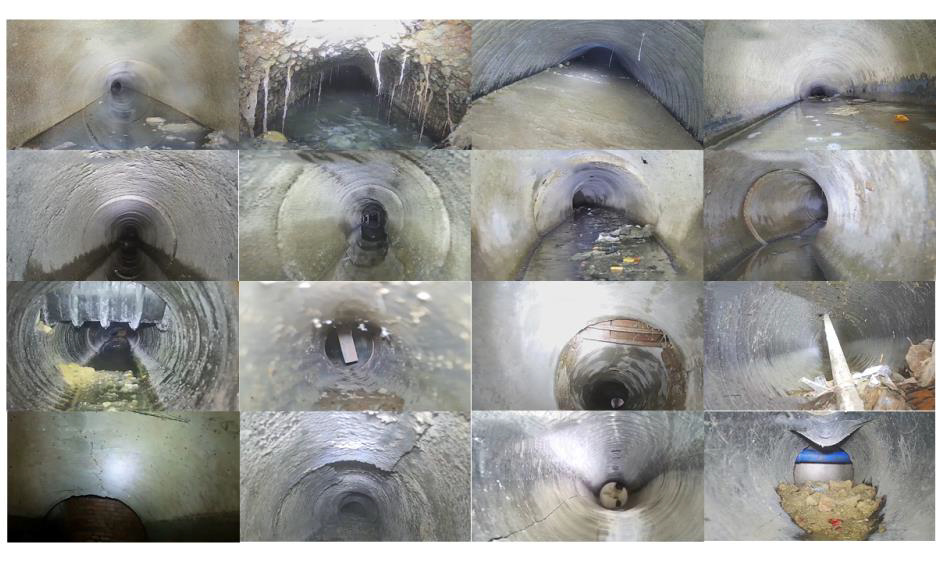
Figure 1. Examples of Our QV-Pipe Dataset
Data Comparison
The QV-Pipe video duration ranges from 0.7 seconds to 385.2 seconds. Each video is annotated by 1 to 5 categories. On average, each video has the duration of 20.7 seconds and 1.4 labels. The 9.6k videos are divided into train set and test set according to the ratio of 2:1. As shown in Figure 2, the data exhibits the natural long-tailed distribution.
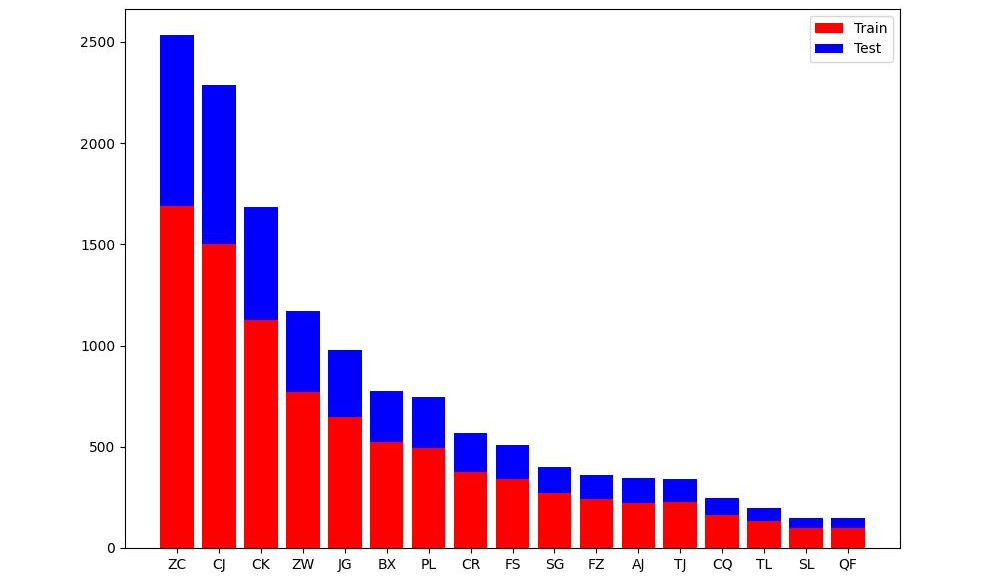
Figure 2. Data Distribution of QV-Pipe
Moreover, we compare it with the existing benchmarks in video anomaly detection. As shown in Table 1, our QV-Pipe dataset shows the following distinct characteristics. First, compared to the existing benchmarks, our QV-Pipe is large scale. Second, each video in our QV-Pipe contains multiple anomaly categories, and these categories are fine-grained. Finally, the previous datasets mainly works on human. Alternatively, the domain shift is large for urban pipe inspection. Hence, our QV-Pipe brings new challenges and opportunities to understand video content for anomaly detection and beyond.
Table 1. Video Anomaly Detection Benchmark Comparison
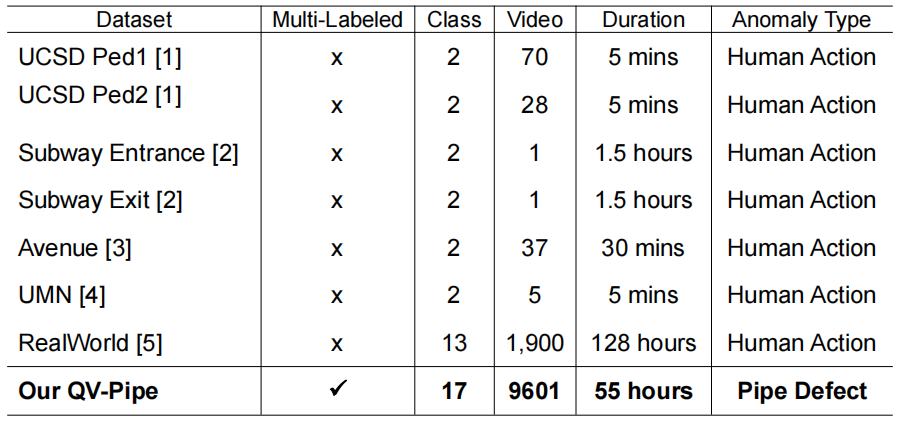
Download
Please refer to the competition page for more information.
Contact Us : Xuan Zhang ( xuan.zhang1@siat.ac.cn )
Reference
- [1] Li, Weixin, Vijay Mahadevan, and Nuno Vasconcelos. "Anomaly detection and localization in crowded scenes." IEEE transactions on pattern analysis and machine intelligence 36.1 (2013): 18-32.
- [2] Adam, Amit, et al. "Robust real-time unusual event detection using multiple fixed-location monitors." IEEE transactions on pattern analysis and machine intelligence 30.3 (2008): 555-560.
- [3] Lu, Cewu, Jianping Shi, and Jiaya Jia. "Abnormal event detection at 150 fps in matlab." Proceedings of the IEEE international conference on computer vision. 2013.
- [4] Raghavendra, R., A. D. Bue, and M. Cristani. "Unusual crowd activity dataset of University of Minnesota." (2006).
- [5] Sultani, Waqas, Chen Chen, and Mubarak Shah. "Real-world anomaly detection in surveillance videos." Proceedings of the IEEE conference on computer vision and pattern recognition. 2018.