Tracks
General description of the problem
Video anomaly analysis is important for industrial applications in the real world. In particular, the urban pipe system is one of the most important infrastructures in a city. In order to ensure its normal operation, we need to inspect pipe defects smartly.
- Quick-View (QV) Inspection is one commonly-used technology. However, it is quite labor-intensive to find defects from a huge number of QV videos. To tackle such problem, we collect a high-quality QV-Pipe dataset and propose this video defect classification task, for automatic, efficient and accurate inspection.
- Closed-Circuit TeleVision (CCTV) is another popular method for pipe defect inspection. Different from short QV videos, CCTV videos are much longer and record more comprehensive content in the very distant pipe. The main task is to discover temporal locations of pipe defects in such untrimmed videos. Clearly, manual inspection is expensive, based on hundreds of hours of CCTV videos. To fill this gap, we proposal a new video benchmark (i.e., CCTV-Pipe) and introduce this temporal localization task for video defect inspection.
Task 1: Video Defect Classification
Predicting the categories of pipe defects in a short QV video.
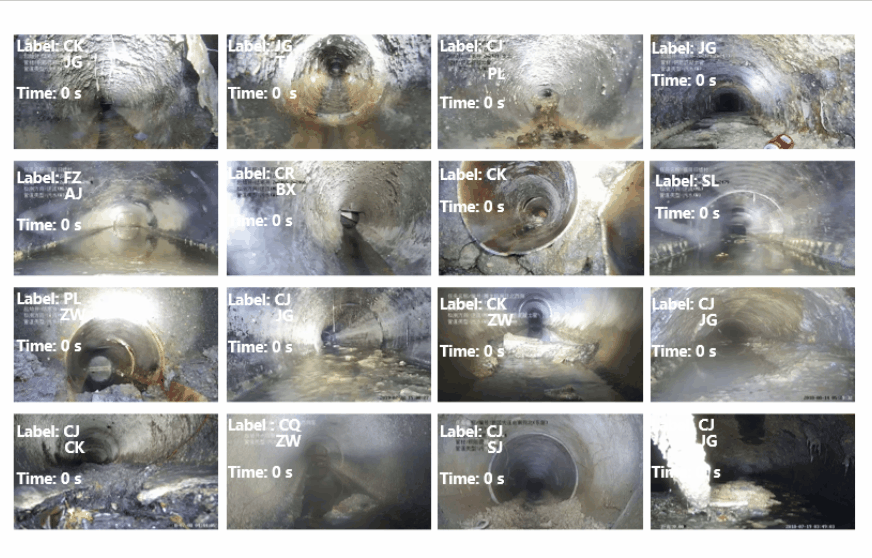
Evaluation Metric
Since each video contains multiple defect categories, we use Average Precision (AP) to evaluate the recognition results on each defect category. Then we average AP over all the categories to obtain mAP.
Please refer to the competition page for more information.
Contact Us : Xuan Zhang ( xuan.zhang1@siat.ac.cn )
Task 2: Temporal Defect Localization
Finding the temporal locations of pipe detects and recognizing their corresponding categories in a long CCTV video.
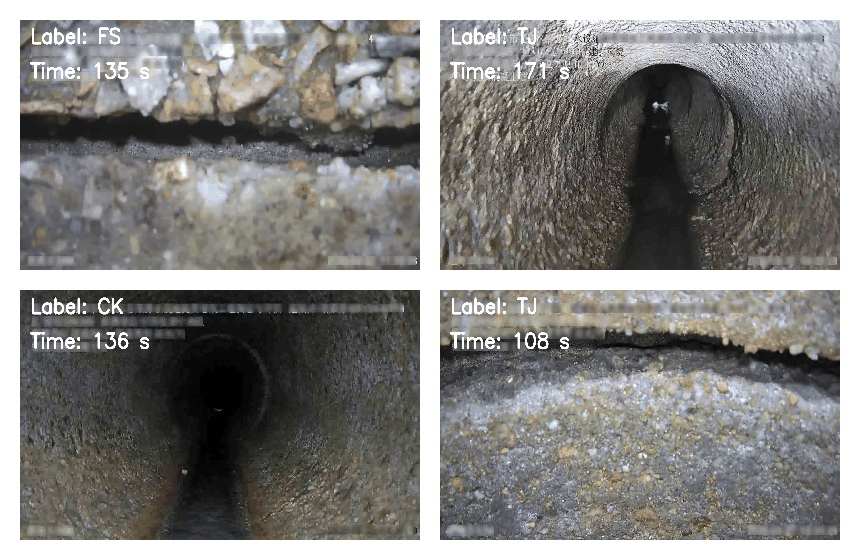
Evaluation Metric
Referring to temporal action localization, we use Average Precision (AP) to evaluate the defect localization results on each defect category. Then we average AP over all the categories to obtain mAP. Due to our single-frame annotations, we compute temporal distance between the predicted defect and the ground truth to check if this prediction is a true positive. Finally, we use the average mAP as evaluation metric, which is the mean of mAP with all the temporal distances (from 1 second to 10 seconds, with 1 second interval).
Please refer to the competition page for more information.
Contact Us : Yi Liu ( yi.liu1@siat.ac.cn )